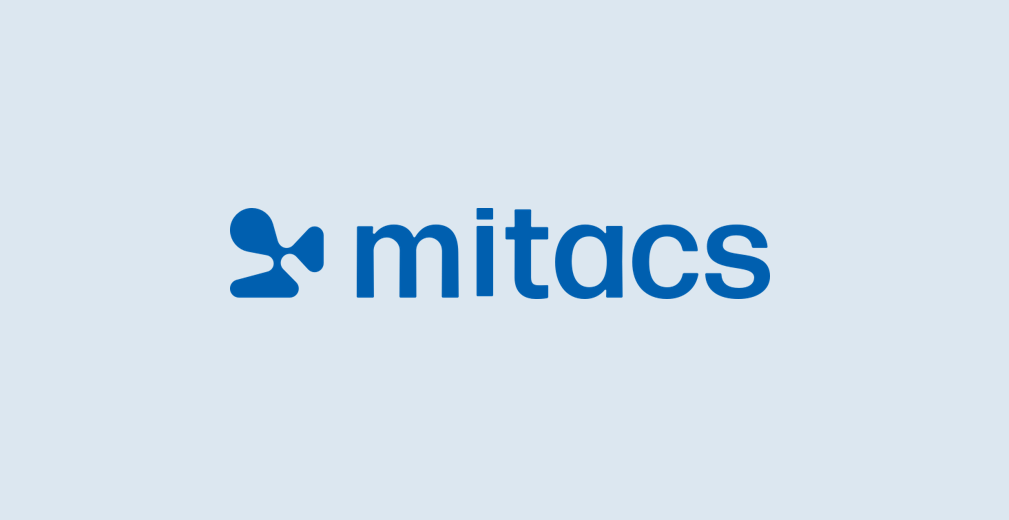
type of treatment they will provide to patients. With technological improvements and the availability of a significant volume of data, it is increasingly difficult for care providers to properly evaluate and analyze the options available to them. The current health condition of the patient–reflected in the monitored observations which are recorded in EMR–may depend on […]
Read More